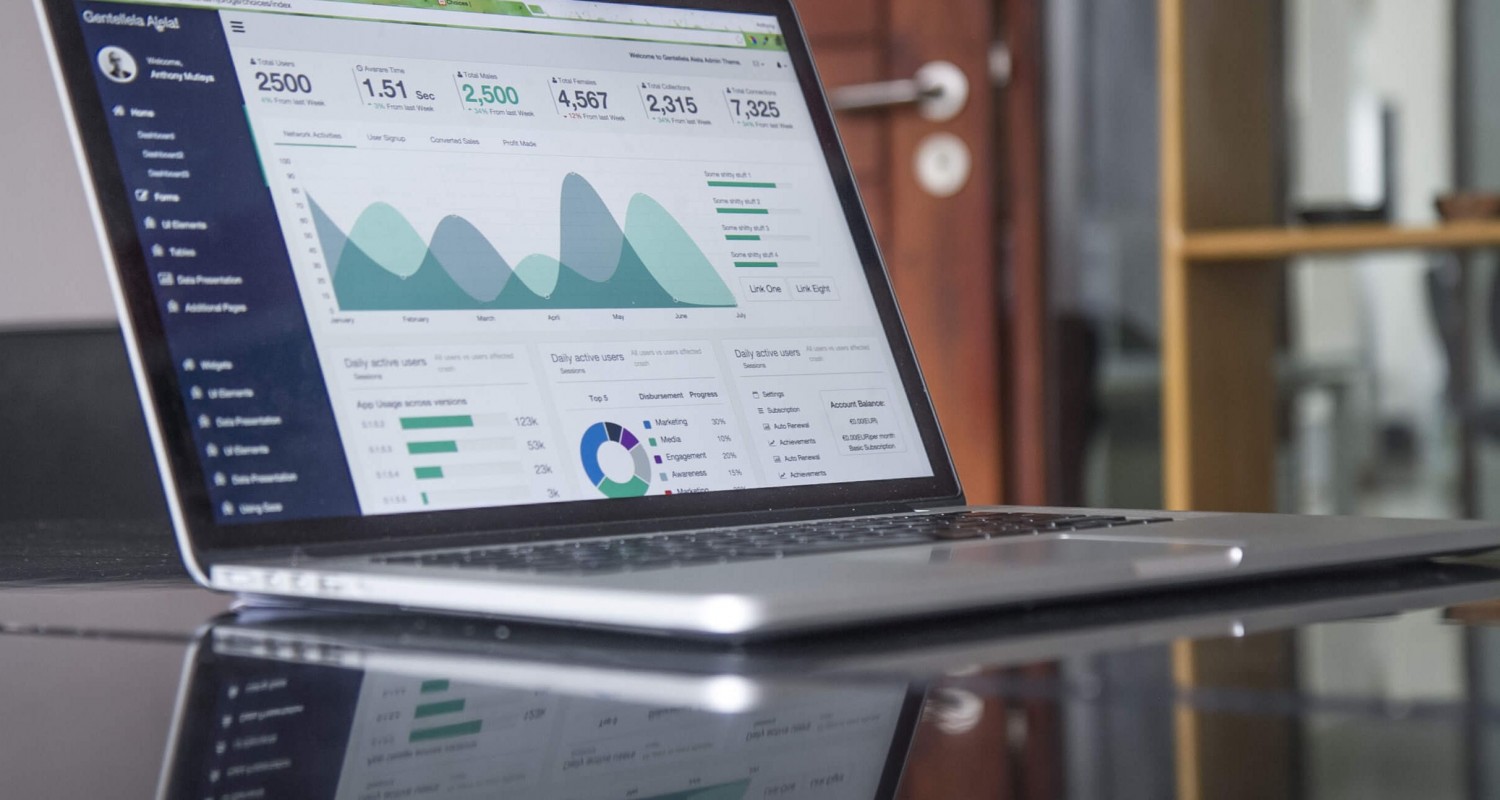
According to Bersin by Deloitte, over the past few years, we have seen incredible growth of HR analytics tools and their adoption rates. In 2018, Bersin reports that there is plenty of extra space for growth but almost a third of companies have reached the highest levels of maturity, becoming “truly digital innovators”.
We at Talent Data Labs wanted to look into these findings to see if, indeed, we are moving towards highly effective talent analytic capabilities or whether we are still throwing mud at a wall and seeing if anything sticks. To do so we researched three key topics:
-
According to decades of research we know that there are four consistently strong predictors of performance (see table 1). But how frequently do we measure them all, and how well do we actually predict them?
-
Do the algorithms out there use a single, multiple, or all four predictive traits of performance (see figure 2)?
-
How valid are the algorithms out there? How many suppliers clearly post and report findings or results similar to what is found in the research?
If you, like us, are curious to learn more about the state of HR analytics then please continue reading below:
The state of HR analytics
The majority of recruitment processes predominantly consider the skill- and job-fit of potential hires, whereas most talent analytics processes account for a variety of other factors as well - such as personality, organisational commitment, cultural fit, and other preferences. This has created a mismatch in available data and required data, which typically prevents us from predicting long-term hiring efficacy. In an ideal scenario, by hiring for the long-term through data and talent analytics we can witness deeper relationships with longer tenure, better referrals, and better employee productivity.
However, it is incredibly laborious and cumbersome to employ data entry or data gathering processes to improve talent analytics competencies. In the post-Big Data era that we live in today, it comes as no surprise that companies are increasingly turning to job matching algorithms to improve the effectiveness and efficiency of their talent acquisition processes. To understand how far we we have come in terms of talent analytics capacities we carried out a detailed comparison and analysis of 20 competitors that utilise job matching algorithms.
1. Out of the four most predictive traits of work performance below, “skills” is most frequently considered
Barring exactly measured scores such as General Mental Ability (GMA), the four traits that we, and over 100 years worth of research (Schmidt & Hunter, 1998), deem most predictive of performance are:
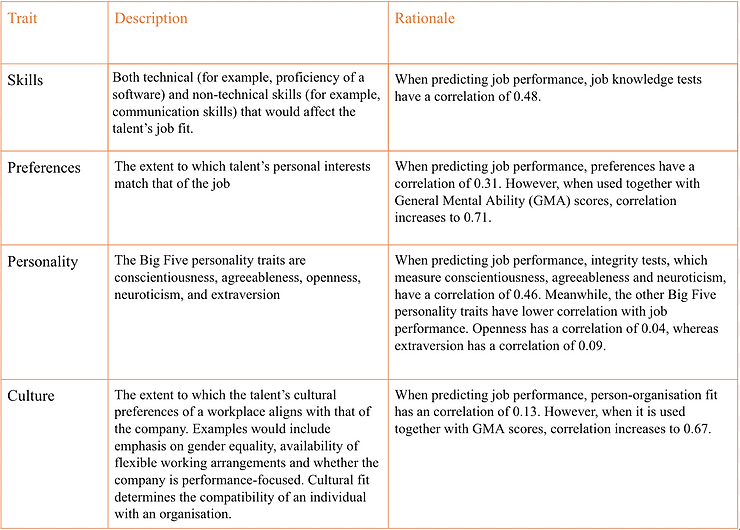
Table 1. Predictive traits: Description and rationale of traits most predictive of performance (Schmidt, Oh & Hunter, 2016)
Hence, we wanted to investigate the percentage of job matching algorithms that consider each factor when calculating the Talent Match scores for a role in a company.
As seen in Figure 1, 95% of algorithms take the skills that potential hires have into account. The second most frequently considered trait is the job and workplace preferences of job candidates (75% of algorithms). 25% of the algorithms incorporate the personality traits of applicants into the matching score. Finally, it is alarming that only 20% of the algorithms that we analysed consider the cultural preferences of candidates since, according to the Society for Human Resource Management (SHRM), hiring someone who is a poor cultural fit can result in turnover costs of 50% to 60% of her annual salary.

Figure 1. Trait integration: Percentage of algorithms that consider the respective predictive traits of performance.
2. Most algorithms consider 2 out of the 4 predictive traits of performance
Ideally, job matching algorithms should account for all 4 predictive traits. We were curious to find out the percentage of algorithms that considered the varying number of predictive traits in the process of generating a Talent Match score. As seen in Figure 2, we found that 70% of the algorithms accounted for 2 predictive traits, while 10% and 15% of algorithms accounted for 1 and 3 traits, respectively. Only a single algorithm took all 4 predictive traits into consideration.
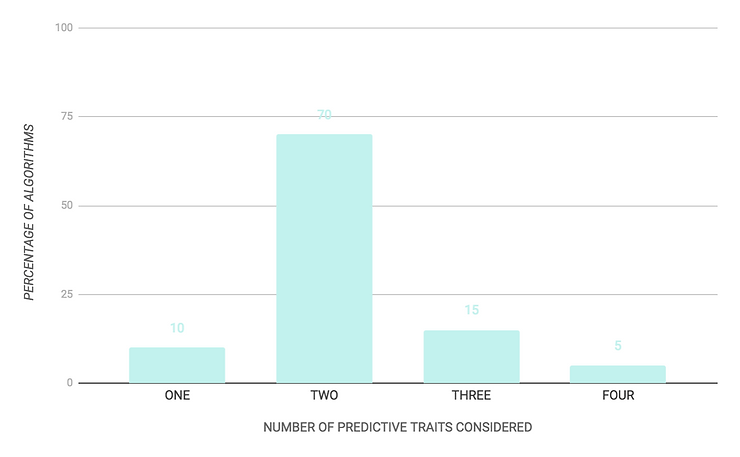
Figure 2. Number of traits integrated: Percentage of algorithms that consider varying number of predictive traits.
3. None of the job matching companies provide access to validation studies
As demonstrated in Table 1, it is clear that the right traits - when measured effectively - are able to predict talent-organisation fit to a great extent. However, what we asked ourselves was whether or not the methodology and findings of the algorithms were supported by an explanation or any sort of transparency. This would make them more reliable and would enable the client to assess the validity of the findings themselves, instead of trusting blindly. We decided to look into this further and were shocked to find that none of the companies investigated provided any access to validation studies. This means that for each Talent Match score provided by these companies the client is simply relying on the word of the company and nothing else.
So what makes Talent Data Labs different?
Here at Talent Data Labs, our tools incorporate all four predictive traits of performance in our Talent Match scores, as we believe that a complete picture should be painted in order to maximise the job and organisational fit of the hire.
We work with clients all over the world; after all, we have regional offices in Europe, America and Asia. Our products are designed with the user-experience in mind, and we automate as many features as possible in order to improve the convenience for our clients.
We offer full transparency for our Talent Match scores, so that you’ll be able understand why certain candidates are more compatible to your organisation. For example, as part of our cultural matching report, we highlight how much a candidate matches or diverges from your company’s culture. Through an interview, you’ll be able to dig further to assess whether the identified diverging factors warrant concern.
"You will be able understand why certain candidates are more compatible with your organisation"
We ensure that our talent matching products are backed by science. Our Cultural Matching score is carefully validated with employee self-reported match, satisfaction and commitment scores, which is supported by studies from Kristof & Brown (2005) and Schmidt and Hunter (1998).
How far have we come?
In conclusion, the state of talent analytics is evolving, but may not be as rose-tinted as Bersin makes us believe. In ignoring valuable information, we may create a tendency to rely excessively on overly simplistic algorithms that help in making predictions but with that fail to truly satisfy our deep desires to eliminate biases and successfully mitigate risk.
-
Due to the difficult and complex nature of the human mind we find that it is hard to use the same practices to measure all different aspects that could predict performance. We therefore focus a lot on measurements that are more widely available and easier to execute through massively available software such as finding skills on LinkedIn.
-
We still close ourselves down for a substantial amount of indicators of performance and focus exceedingly narrow on one or two traits that we deem most important ignoring the others.
-
If we truly did talent analytics right we would expect reports from all over the world of people now more accurately predicting tenure, performance, promotions, growth, and other outcome variables. Unfortunately these findings are rare and usually rely on face validity thus ignoring our desires to prevent bias.
Reading the reports of Bersin we might believe that Talent Analytics has reached a certain state of maturity. However, when we do our research we find that there is a lot of missing evidence to support such a statement. Luckily, there is hope! Thanks to all the new tools and great products that are being developed out there, the path ahead of us to widely deployed improved predictions is quite clear.
We at Talent Data Labs aim to help all of you navigate those roads effectively, so please do reach out to us if you found this analysis compelling and want to work on a solution with us!